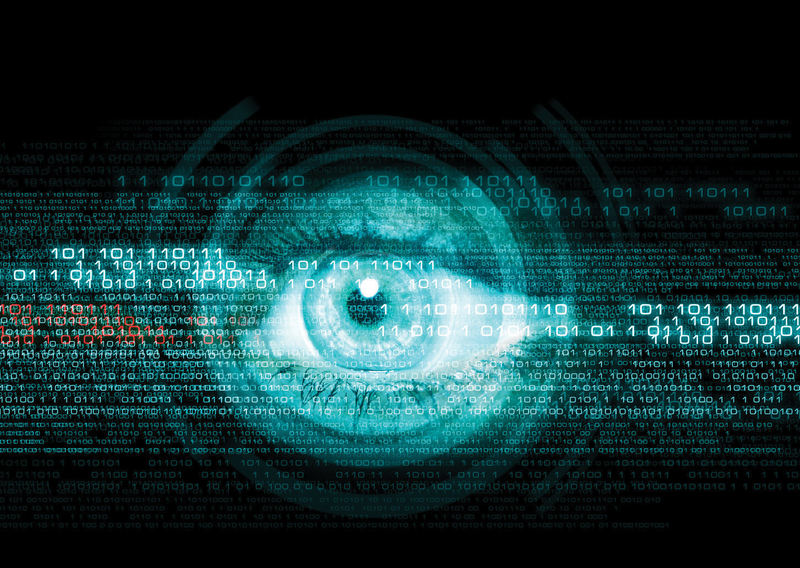
PostgreSQL 9.5: What's new; what's better?
An Eye for Details
PostgreSQL 9.5 contains more than 100 changes, including dozens of performance improvements. The first highlight in PostgreSQL 9.5 is the extension of the INSERT
command to include the ON CONFLICT DO UPDATE
clause, which lets you stipulate that an UPDATE
is performed instead of an INSERT
if the line to be inserted already exists. This behavior is also known as "UPSERT."
UPSERT
In practical applications, the UPSERT case occurs quite often, but users of PostgreSQL previously had to implement the solution manually as a stored procedure or rely on the logic in the client application. The difficulty originates with the defaults in the SQL standard and other database systems, which cause considerable technical problems unacceptable to the PostgreSQL developers [1]. Although the logic of an UPSERT is quite simple, it becomes complicated if this logic needs to deliver high performance in parallel operations without generating deadlocks or sporadic, unique constraint errors, which is very much the case with other implementations.
For these reasons, the PostgreSQL project decided specifically to design a separate syntax with its own semantics. In this example, a table stores subscriptions from a service with a user ID, the subscription type, and the start and end dates:
CREATE TABLE subscriptions ( user_id int NOT NULL, subscription_type text NOT NULL, started date NOT NULL, expires date NOT NULL, PRIMARY KEY (user_id, subscription_type) );
An UPSERT operation is now required to either insert a new entry or update an existing entry. Previously, developers needed to rely on a loop that performed INSERT
or UPDATE
operations until one of them worked without error. You could do this, for example, with a PL/pgSQL function of the type shown in Listing 1. The loop is necessary in this example because another connection could add a conflicting line between the UPDATE
and the INSERT
.
Listing 1: UPSERT Replacement
01 CREATE FUNCTION set_subscription(in_user_id int, in_subscription_type text, in_started date, in_expires date) RETURNS void 02 LANGUAGE plpgsql 03 STRICT 04 AS $$ 05 BEGIN 06 <<upsert>> 07 LOOP 08 UPDATE subscriptions 09 SET (started, expires) = (in_started, in_expires) 10 WHERE (user_id, subscription_type) = (in_user_id, in_subscription_type); 11 EXIT upsert WHEN FOUND; 12 BEGIN 13 INSERT INTO subscriptions (user_id, subscription_type, started, expires) 14 VALUES (in_user_id, in_subscription_type, in_started, in_expires); 15 EXIT upsert; 16 EXCEPTION WHEN unique_violation THEN 17 END 18 END LOOP; 19 $$;
The logic of Listing 1 can be shortened to a single command with an UPSERT, as shown in Listing 2. The command initially comprises a normal INSERT
, followed by an ON CONFLICT
clause describing the conflict and the action to be performed. In this typical case, the conflict relates to the potential ambiguity of the primary key as soon as an attempt is made to insert the same user with the same subscription once again. The action is an UPDATE
command.
Listing 2: With UPSERT Command
01 INSERT INTO subscriptions (user_id, subscription_type, started, expires) 02 VALUES (in_user_id, in_subscription_type, in_started, in_expires) 03 ON CONFLICT (user_id, subscription_id) 04 DO UPDATE SET (started, expires) = (in_started, in_expires);
As you can see, the new command saves a great deal of code and offers better performance by avoiding the problem of DIY implementations, which need to be anticipated for cases in which the action fails in other connections.
If you want to avoid repeating the input values from the VALUES
clause in the UPDATE
command, you can also use the EXCLUDED
alias to point to the value of the line whose insert failed (Listing 3). On the other hand, you can also write completely different values in the UPDATE
than in the INSERT
if you somehow want to log the fact that a conflict occurred.
Listing 3: With EXCLUDED
01 INSERT INTO subscriptions (user_id, subscription_type, started, expires) 02 VALUES (in_user_id, in_subscription_type, in_started, in_expires) 03 ON CONFLICT (user_id, subscription_id) 04 DO UPDATE SET (started, expires) = (EXCLUDED.started, EXCLUDED.expires);
Although UPSERT is more likely to be useful to developers of web applications and other transactional applications, PostgreSQL 9.5 also shows innovations in the field of analytics.
Group Theory
Listing 4 shows some sample data and a number of applications of the grouping function. PostgreSQL supports grouping operations known from other SQL systems, as well, such as CUBE
and ROLLUP
, which extend the well-known GROUP BY
clause and support the execution of multiple grouping variants at the same time.
Listing 4: New Grouping Options
01 CREATE TABLE HR ( 02 country text, 03 city text, 04 department text, 05 name text 06 ); 07 08 INSERT INTO HR VALUES 09 ('DE', 'Berlin', 'Sales', 'Christian'), 10 ('DE', 'Berlin', 'Marketing', 'Sandra'), 11 ('DE', 'Frankfurt', 'Sales', 'Stefan'), 12 ('AT', 'Innsbruck', 'HR', 'Katrin'), 13 ('CH', 'Zurich', 'Sales', 'Alexander'); 14 15 SELECT country, count(*) FROM HR GROUP BY country; 16 SELECT country, city, count(*) FROM HR GROUP BY country, city; 17 SELECT department, count(*) FROM HR GROUP BY department; 18 SELECT country, department, count(*) FROM HR GROUP BY country, department;
CUBE
or ROLLUP
let you combine the scan queries. Listing 5 shows an example using ROLLUP
. The option groups on the specified columns – as a normal GROUP BY
would – and by all prefixes of the column list, including the empty list. This is particularly useful for hierarchical structures. In the example, you can see figures at the local departmental level and at the superordinate organizational levels, as well as the total number for each query. Without ROLLUP
, you need to do this with separate queries (or possibly glue them together with a UNION
), which would be troublesome and slower.
Listing 5: An Example with ROLLUP
> SELECT country, city, department, count(*) FROM HR GROUP BY ROLLUP (country, city, department) ORDER BY 1, 2, 3; +---------+-----------+------------+-------+ | country | city | department | count | +---------+-----------+------------+-------+ | AT | Innsbruck | HR | 1 | | AT | Innsbruck | | 1 | | AT | | | 1 | | CH | Zurich | Sales | 1 | | CH | Zurich | | 1 | | CH | | | 1 | | DE | Berlin | Marketing | 1 | | DE | Berlin | Sales | 1 | | DE | Berlin | | 2 | | DE | Frankfurt | Sales | 1 | | DE | Frankfurt | | 1 | | DE | | | 3 |
The CUBE
option groups by other possible combinations of the grouping columns. In the results for the example in Listing 6, you can see the global totals for the various departments. However, not all of the lines from the CUBE
results make sense in this example. The NULL, Berlin, NULL line, for example, groups all cities by the name of Berlin in all countries. This may have some attraction as a curiosity, but very little practical use.
Listing 6: An Example with CUBE
> SELECT country, city, department, count(*) FROM HR GROUP BY CUBE (country, city, department) ORDER BY 1, 2, 3; +---------+-----------+------------+-------+ | country | city | department | count | +---------+-----------+------------+-------+ | AT | Innsbruck | HR | 1 | | AT | Innsbruck | | 1 | | AT | | HR | 1 | | AT | | | 1 | | CH | Zürich | Sales | 1 | | CH | Zürich | | 1 | | CH | | Sales | 1 | | CH | | | 1 | | DE | Berlin | Marketing | 1 | | DE | Berlin | Sales | 1 | | DE | Berlin | | 2 | | DE | Frankfurt | Sales | 1 | | DE | Frankfurt | | 1 | | DE | | Marketing | 1 | | DE | | Sales | 2 | | DE | | | 3 | | | Berlin | Marketing | 1 | | | Berlin | Sales | 1 | | | Berlin | | 2 | | | Frankfurt | Sales | 1 | | | Frankfurt | | 1 | | | Innsbruck | HR | 1 | | | Innsbruck | | 1 | | | Zürich | Sales | 1 | | | Zürich | | 1 | | | | Marketing | 1 | | | | HR | 1 | | | | Sales | 3 | | | | | 5 |
If you want even more control over the output, you can use a GROUPING SETS
clause to specify the combinations to be grouped. After all, CUBE
and ROLLUP
themselves are simply abbreviations for specific GROUPING SETS
. The example in Listing 7 shows a potential use case.
Listing 7: GROUPING SETS
> SELECT country, city, department, count(*) FROM HR GROUP BY GROUPING SETS ((country, city, department), (country, city), (country), (), (department), (country, department)) ORDER BY 1, 2, 3; +---------+-----------+------------+-------+ | country | city | department | count | +---------+-----------+------------+-------+ | AT | Innsbruck | HR | 1 | | AT | Innsbruck | | 1 | | AT | | HR | 1 | | AT | | | 1 | | CH | Zürich | Sales | 1 | | CH | Zürich | | 1 | | CH | | Sales | 1 | | CH | | | 1 | | DE | Berlin | Marketing | 1 | | DE | Berlin | Sales | 1 | | DE | Berlin | | 2 | | DE | Frankfurt | Sales | 1 | | DE | Frankfurt | | 1 | | DE | | Marketing | 1 | | DE | | Sales | 2 | | DE | | | 3 | | | | Marketing | 1 | | | | HR | 1 | | | | Sales | 3 | | | | | 5 |
A Little More Partitioning
PostgreSQL has no built-in solution to partitioning, and this does not change in PostgreSQL 9.5. The typical workaround is to construct your own partitioning manually. An inheritance hierarchy is a useful solution (Listing 8).
Listing 8: DIY Partitioning
> CREATE TABLE logins (tx timestamp, ip_adress, ...); > CREATE TABLE logins_201509 (CHECK (tx >= '2015-09-01' AND tx < '2015-10-01')) INHERITS (logins); > CREATE TABLE logins_201510 (CHECK (tx >= '2015-10-01' AND tx < '2015-11-01')) INHERITS (logins);
Using a combination of triggers and constraints, you can divide the data up into various physical tables, and the user only needs to cite the underlying parent table when reading. The database automatically accesses the matching child tables. Some PostgreSQL extension modules can help establish and manage these structures with less manual overhead – pg_partman
being one example.
One innovation in PostgreSQL 9.5 is that the subordinate child tables can also be foreign tables that reside on other computers. Foreign tables are tables the database accesses via plugins known as foreign data wrappers (FDWs), which can be data from other database systems, from files, and even from Internet services. Another PostgreSQL database is also an option.
Foreign tables can now have CHECK
constraints. In partitioned tables, the planner looks at the constraints in the database to skip the partitions whose CHECK
constraints rule out the query criteria. The combination of these two innovations thus makes it possible to use the same approach as previously for partitioning but also extend partitioning beyond computer boundaries.
Sampling
One focus of the work on PostgreSQL 9.5 was to improve the way it handles very large tables. One contribution is the TABLESAMPLE
feature, which is known from the SQL standard and a number of commercial databases. It lets programmers simply read a random selection of lines from a table. Therefore, queries against very large tables can be substantially accelerated if approximate results are sufficient.
For example, you could use the approximate average price of all articles on the system:
SELECT avg(preis) FROM article TABLESAMPLE SYSTEM(1);
The statement only reads 1% of the lines in the article
table and uses the SYSTEM
random sampling method. This method reads randomly selected blocks of the table and omits all others. Alternatively, you could use the BERNOULLI
method, which is slower because it needs to read the entire table – although the random selections are superior. In typical PostgreSQL style, you can define your own random sampling methods using extensions.
Indexing
A new BRIN (Block Range Index) indexing method is designed for very large tables. A BRIN stores the minimum and maximum values for each block range. When queried with a range query (e.g., x < 10
), it only needs to consider those blocks whose boundary values include the sought-after range. Although this is not as efficient as the well-known B-tree, which points directly to the matching rows, because BRIN only points to a block range, a BRIN is much smaller than a legacy B-tree index.
For example, a table with an int4
column populated with the numbers 1 through 100 million is about 3.5GB. The B-tree index for this would take about 2.1GB, whereas a BRIN only occupies 100KB. The benefit of BRINs is particularly felt in tables that are far larger than the amount of available RAM, and where even the B-tree indexes would be too large for RAM.
BRIN is best suited to data that are naturally stored in ascending order (e.g., timestamps in a continuous process or automatically generated identification numbers). In this case, the BRIN can be used in a query such as time > 'yesterday'
; it skips large parts of the table because only very few contiguous blocks contain the matching data. However, BRIN is pretty much useless for randomly distributed values because more or less all the block ranges contain matching values, and an index scan is pretty much the same as a sequential scan.
Row-Level Security
The system of access permissions in SQL only defines access at the table or column level because these are the units envisaged for this purpose by the Data Definition Language (DDL). In some applications, managing access to individual rows of data is interesting and can be done manually, as is so often the case in PostgreSQL, using special views and triggers.
Now in PostgreSQL 9.5, you have a simple, built-in solution. Listing 9 demonstrates a typical use case, wherein each row stores the user who created the row. Reading the table then only shows the rows that belong to the user in question. This means that every member of staff can manage the data for which they are responsible, but the programmer does not have to worry about giving users undesirable access to data.
Listing 9: Row-Level Access Controls
> CREATE TABLE orders ( id int, product text, number int, employee text ); > ALTER TABLE orders ENABLE ROW LEVEL SECURITY; > CREATE POLICY p1 ON orders FOR ALL TO PUBLIC USING (employee = current_user); > GRANT ALL ON TABLE orders TO PUBLIC; > SET SESSION AUTHORIZATION m1; > INSERT INTO orders VALUES (1, 'Screw', 10, 'm1'); > INSERT INTO orders VALUES (2, 'Nail', 5, 'm2'); -- <Error: not allowed> > SET SESSION AUTHORIZATION m2; > INSERT INTO orders VALUES (3, 'Hammer', 1, current_user); > SET SESSION AUTHORIZATION m1; > SELECT * FROM orders; +----+----------+--------+-------------+ | id | product | number | employee | +----+----------+--------+---------- --+ | 1 | Screw | 10 | m1 | +----+----------+--------+-------------+ > SET SESSION AUTHORIZATION postgres; > SELECT * FROM orders; +----+----------+--------+-------------+ | id | product | number | employee | +----+----------+--------+-------------+ | 1 | Screw | 10 | m1 | | 3 | Hammer | 1 | m2 | +----+----------+--------+-------------+
Data to which access is not granted are practically invisible. Row-level security thus differs from legacy privilege management systems that throw an error with missing access privileges. With this system, you can, for example, simply implement a multitenancy system, which means an application can be provided to multiple, separate customers who use the same table internally – without running the risk of one customer seeing another customer's data.
Previously, you had to use separate tables to achieve this, but it could easily have caused problems if you had many thousands of tables, and the only alternative would have been an implementation based on troublesome and error-prone triggers and views.
pg_rewind for Admins
Database administrators will find something new in the replication area. For example, assume that a master server has failed and a failover to the slave has taken place. Later, the master server is restored. Previously, it was typically impossible to join the previous master as a slave to the new master because the write-ahead log timelines were divergent. This only worked if you shut down the master gracefully before shutting down the slave, which is not an option in a crash.
The only possibility was to delete the data on the old master and build a slave completely from scratch, which could be very time consuming. During the process, the database did not have a slave, except in cases for which a redundant slave existed. A new program, pg_rewind
, now makes it possible to convert the former master into a functional slave:
pg_rewind -D /usr/local/pgsql/data --source-server='host=db2 dbname =postgres'
The program runs the previous master and edits the specified data directory so that it can be appended to the specified new master, which can be running on a different computer. Subsequently, the previous master can then be restarted using a recovery.conf
file.
Typos
Finally, an innovation for everyone who enjoys working at the command line. If you are familiar with Git's ability to detect typos and make suggestions for correcting them, PostgreSQL also now has that faculty:
test=> select citx, country from HR; \ ERROR: column "citx" does not exist \ LINE 1: select citx, country from HR; \ HINT: Perhaps you meant to reference the column "HR"."city".
However, this currently only works for column names.
Conclusions
PostgreSQL is a community project without centralized commercial management. The features in each version are not defined by a release plan or roadmap. They come from developers working for different companies and with different plans. Nevertheless, you can still identify trends. One focal topic in PostgreSQL 9.5 was support for large databases with analytics applications, and you can expect more of the same in the next few versions. Additionally, the UPSERT feature fills a function gap in typical web applications.
The upgrade is well worthwhile – even if you do not need the new functions.