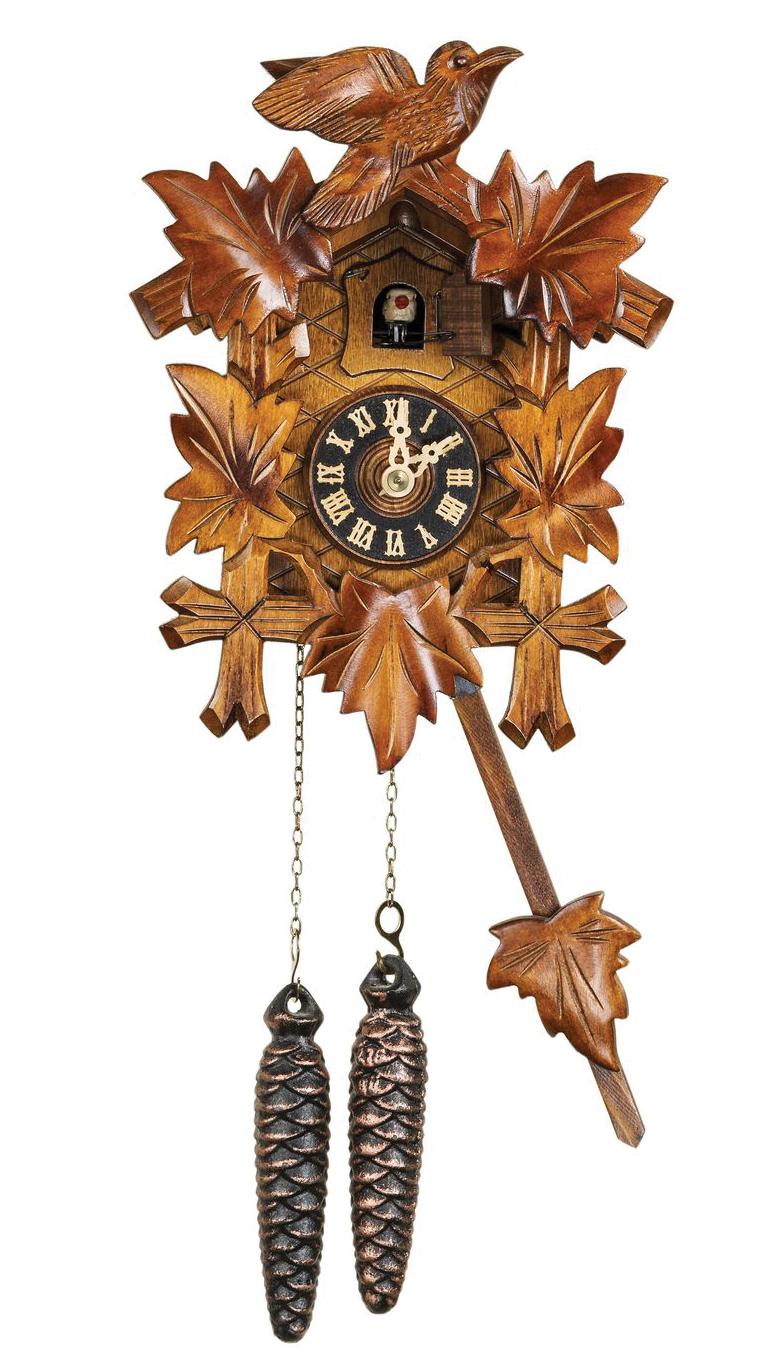
The Cuckoo sandboxing malware analysis tool
Cuckoo, Cuckoo
Security researchers and local computer emergency response teams try to understand what exactly malicious software does and what communication channels to external systems it relies on. Ultimately, they have two basic ways to draw conclusions about where the software came from and its intentions: reverse engineering and sandboxing. The tool presented here, Cuckoo, is a sandboxing tool.
Gaining Access
With ransomware and similar forms of malware, the modus operandi of the attacker is typically predictable: To begin, they need to find a gateway into the target network. Other than exploiting vulnerabilities in software, email malware is a very popular way to breach a network, with a recent trend – that unfortunately outsmarts some mail scanners – of adding multiple attachments, with only one attachment containing the malicious software. Social engineering also plays an important role in acquiring information that serves as the basis for an attack.
Once an attacker finally gains access to a system and dumps the malware, the next step usually is to install a remote access tool (RAT) that is handled by a command and control system. At this stage, an attacker can establish permanent access to the infected system and other systems on the local network to get to the desired data.
Malware Analysis Methods
To apply reverse engineering, you examine and disassemble the software statically to understand what the code does, which requires appropriate tools (e.g., the well-known IDA Pro [1]). For sandboxing, you install the software within a secure environment, often a virtual machine or hardware appliance (e.g., FireEye [2] is a well-known manufacturer of such appliances), and observe its activities in real time.
Both methods have their advantages and disadvantages. Reverse engineering requires a very good understanding of machine language to deal with the appropriate tools and interpret the results, but it does give deep insights into the code of the software and the ability to explore the tiniest nooks and crannies. Sandboxing produces much faster results, with the risk, however, that the malware detects it is running in a sandbox and thus potentially executes completely different code paths than it would outside such an environment. The disadvantage of hardware appliances is that they are less flexible than software, which you can adapt to suit your own landscape.
Cuckoo [3] was launched in August 2010, and the release candidate for version 2.0 was published recently. The Linux software is licensed under the GPL3 and is available for download free of charge. Although Cuckoo is used in professional environments, the freely available software is interesting for students and young researchers who tend to shy away from investing in what are often very expensive hardware appliances. Cuckoo operates parallel to hardware appliances in part, because the software's modular approach lets you adjust it for your own environment and thus achieve better test results.
Cuckoo offers a number of interesting features: The software analyzes a wide variety of file types and monitors every system call to the malicious software running inside a virtual machine. It observes all files that are created, deleted, or loaded from external sources by the malware; records network traffic and saves a dump as a PCAP trace for evaluation; and creates a memory dump of both the complete virtual machine and of the malware processes to secure the contents of volatile memory. If you pick up the special wget.py
module for downloading malware [4] from the Cuckoo Git repository and copy it to the install folder /analyzer/linux/modules/packages/
, you can also examine entire websites for malware [5]. All the results are summarized in a report and staged for evaluation.
Architecture
At Cuckoo's heart is a central management component that is responsible for scheduling analyses and evaluating results. The jobs themselves run on isolated virtual machines that are newly generated for each analysis task (Figure 1). Cuckoo requires a Linux host system, although the software probably has also been used successfully on Mac OS. The supported virtualization solutions are VMware, VirtualBox, and even KVM/libvirt. Within the virtual systems on which the malware is installed, Cuckoo supports Windows, Mac OS, Linux, and Android.
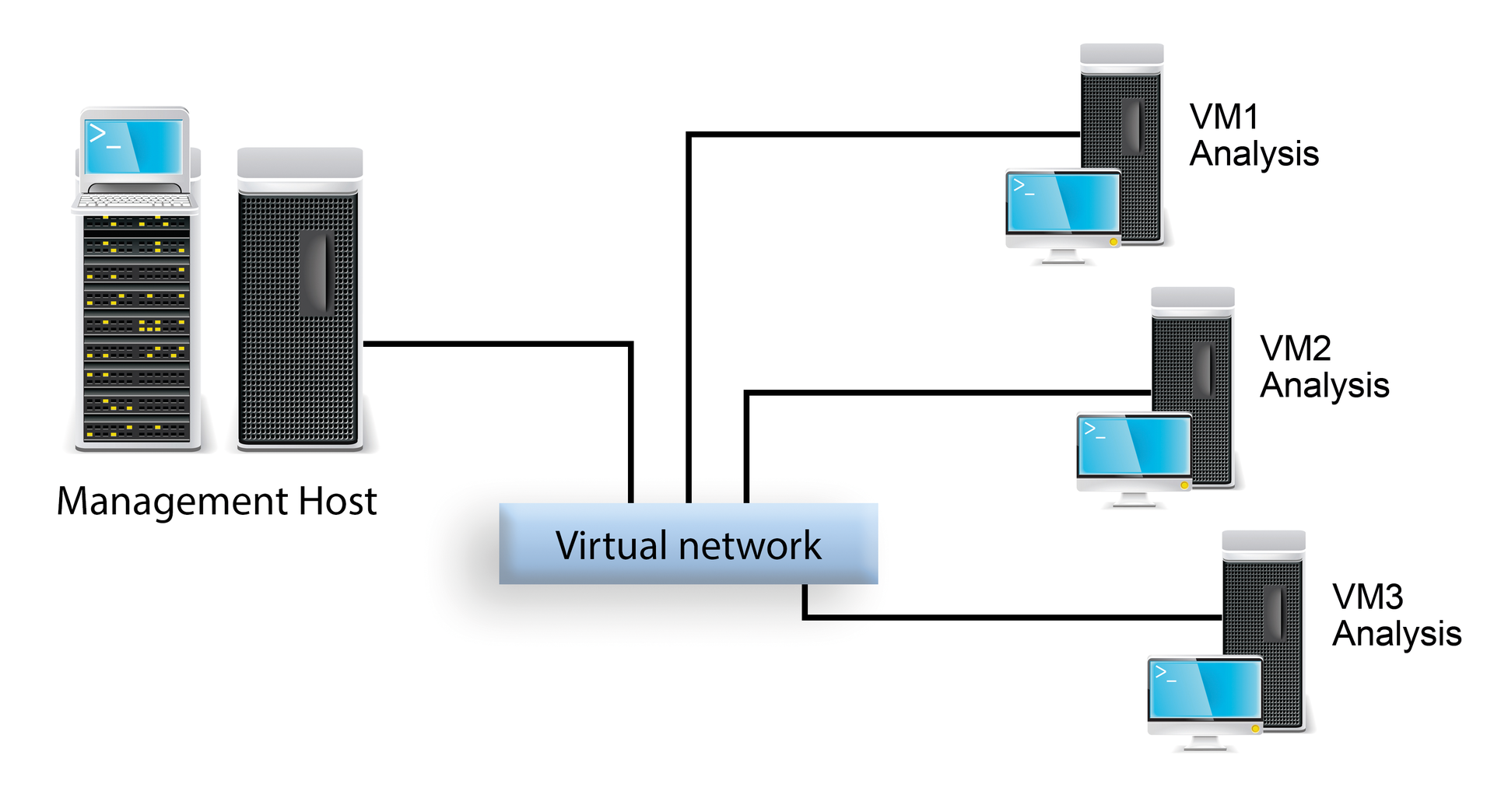
Installation
The examples in this article are all based on an installation of Cuckoo 2.0 RC1 on Fedora 23 and KVM/libvirt. The archive with the sandboxing software is available for download [3]. After unpacking, you need to create a cuckoo user and add the account to the libvirt group. Because Cuckoo must at all times be in a position to create a virtual machine using the libvirt framework, the Polkit rule from Listing 1 ensures that access to the framework is possible for all members of the libvirt group.
Listing 1: Polkit Rule for libvirt
polkit.addRule(function(action, subject) { if (action.id == "org.libvirt.unix.manage" && subject.isInGroup("libvirt")){ return polkit.Result.YES;} });
In the conf/
folder, you will want to check out the configuration files, of which there are many, including cuckoo.conf
, kvm.conf
, auxiliary.conf
, memory.conf
, processing.conf
, and reporting.conf
. For a first test, the cuckoo.conf
, auxiliary.conf
, and kvm.conf
files are the most important. If you use any virtualization solution other than KVM/libvirt, such as VMware or VirtualBox, suitable configuration files are available for them, too.
Basic settings for operating Cuckoo are defined in the cuckoo.conf
file. For example, a few of the parameters you can use, all of which are very well documented, define which virtualization solution to use (e.g., machinery = kvm
), the host to which reports and logfiles are sent (ip
), and whether a memory dump file of the virtual machine is to be created (memory_dump
).
If you use KVM/libvirt on the management system, you need to customize the settings in the kvm.conf
file to ensure that the name (machines
), label, IP address (ip
), and operating system (platform
) of the virtual machine are specified correctly. Of course, several machines can be defined at this point, because all statements within a separate section apply to just this machine. If you have, say, virtual machines named fed01 and win01, then the entries in the kvm.conf
file might look like Listing 2.
Listing 2: Sample Configuration
machines = fed01, win01 interface = virbr0 [fed01] label = fed01 platform = linux ip = 192.168.122.10 [win01] label = win01 platform = windows ip = 192.168.122.110
Additional services can be integrated from the auxiliary.conf
file. For example, this is where you determine whether to dump the virtual machine's network traffic. The services are implemented via a separate module in the modules/auxiliary/
folder and can be extended if necessary. Generally, this customization approach applies to all configurations in Cuckoo and is one of the great strengths of the software.
The memory.conf
file defines what type of tests to perform on the memory dump of the virtual machine. For example, you can define whether the memory should be searched for specific kernel modules, and you can specify in the processing.conf
file what exactly the analysis of the malware samples should look like. Among other things, integration with the VirusTotal online service is possible, or you can specify that a Python script is generated dynamically based on the malware process dump; the script can then be download for further analysis in IDA Pro. Finally, the entries in the reporting.conf
file determine what form the Cuckoo reports should take. The JSON format is a useful choice for automated processing of the results downstream. HTML reports are fine if you want an overview of the analysis results. If you team Cuckoo with MongoDB, you can use the Django-based web interface to access the results.
Even though we have not yet created a virtual machine to analyze the malware samples, Cuckoo should launch with these settings. The call to ./cuckoo.py
from the installation directory welcomes the user with a nice display of ASCII art (Figure 2). You still can't do much, because the virtual machines that Cuckoo uses as the basis for analyzing the malware samples we will be passing in and their snapshots need to be generated in the next step.
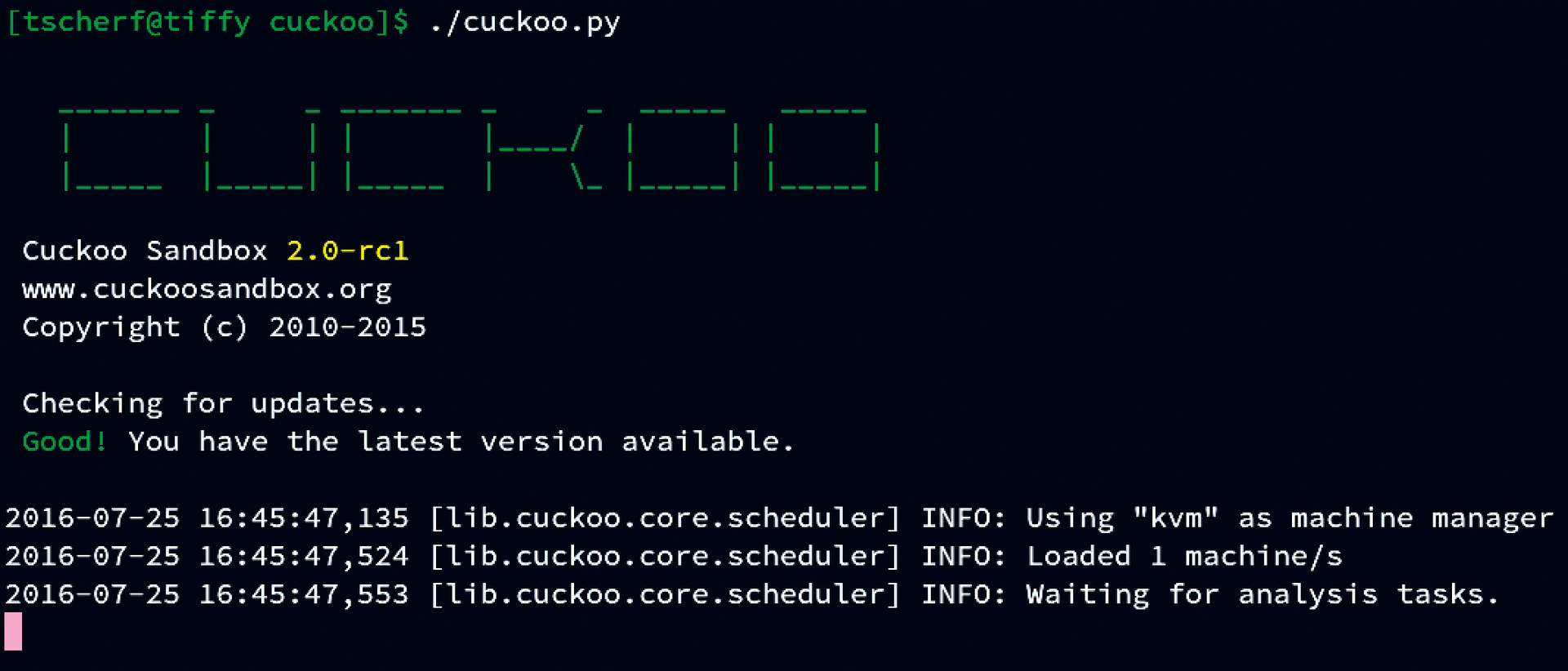
Generating Machine Templates
Cuckoo does not have its own procedure for generating virtual machine templates; instead, it relies on existing tools and mechanisms. For this article, I used a single virtual machine based on Fedora. You can use the graphical virt-manager
tool for the installation, or virt-install
in the shell. The connection to the host system running the Cuckoo Management Framework is controlled by a bridge. KVM/libvirt uses the private IP address space 192.168.122.0/24, where the address 192.168.122.1 is assigned to the host system. Hard drives should be created as LVM or QCOW2 volumes within the virtual machine; otherwise, no snapshots of the machine can be generated. A description of the complete installation is beyond the scope of this article, which is why I refer you to the existing installation instructions [6]. You also need to ensure that Python 2.7 is installed on the virtual machine, because the Cuckoo analysis software requires this version.
Assuming that the installation of the machine is successful, you should update the kvm.conf
configuration file on the Cuckoo host system with the correct data for the machine. As already mentioned, this includes the IP address, the name, and the label of the virtual system. Finally, you need to copy to the machine the Cuckoo agent (agent.py
), which you will find on the host system in the Cuckoo installation folder agent/
.
You can start the agent on the virtual machine with the help of the shell script also found in the same folder. The folder in which the agent is stored doesn't really matter. The agent implements an XMLRPC server that waits for incoming connections from the host system. The malware samples are then sent to the system through these connections. Ensure that the agent starts automatically after rebooting the virtual machine before creating a snapshot of the machine in the next step. You can create such a snapshot using libvirt's own tools:
# virsh snapshot-create fed01 # virsh snapshot-list fed01 Name Creation Time State ----------------------------------- 1469460006 2016-07-25 [...] running
To avoid problems, only one snapshot should ever exist per virtual machine. After the snapshot is created, the virtual machine can be turned off. Cuckoo will now access the system's snapshot as soon as a sample is received and analyzed within a virtual system instance.
At this point, note that existing virtual systems can also serve as a basis for Cuckoo. If you already have such a system and only need to change the disk types, you can easily convert such a image with the command:
# qemu-img convert -O qcow2 fed01.raw fed01.qcow2
Then you need to create the new disk type (<driver name='qemu' type='qcow2'/>
) in the XML definition of the virtual machine and state the storage location of the image file (<source file='/var/lib/libvirt/images/fed01.qcow2'/>
). The easiest way to do this is to use the command:
# virsh edit fed01
Again, replace the fed01
label with the label of your own virtual machine, save the file, and start the virtual system; it should be possible to create a snapshot.
Cuckoo Operation
For a first test, your best bet is to use the European Institute for Computer Antivirus Research (EICAR) test file, which is detected as a virus by most malware analysis systems [7]. To keep the file name from triggering Cuckoo, I renamed the file readme.txt
for my tests.
Several options allow you to send the fake malware to Cuckoo (e.g., the Django web interface lets you upload files). Cuckoo itself offers a feature-rich API that lets you send malware samples to the management system from your own applications. The easiest approach, though, is to use submit.py
from Cuckoo's utils
folder. The tool has many options, but in the simplest case, calling it with the file path of the malware as a parameter will suffice:
$ utils/submit.py tests/readme.txt Success: File "/home/tscherf/cuckoo/test/readme.txt" added as task with ID 6
For Cuckoo to accept the file, the management framework must be started up front by running python cuckoo.py
from the installation folder – if you have not done this already. Immediately after posting a sample, Cuckoo outputs appropriate messages on the console (Listing 3).
Listing 3: Cuckoo Output
2016-07-25 17:37:00,192 [lib.cuckoo.core.scheduler] INFO: Starting analysis of FILE "readme.txt" (task #6, options "") 2016-07-25 5:37:00 PM,207 [lib.cuckoo.core.scheduler] INFO: File already exists at "/home/tscherf/cuckoo/cuckoo/storage/binaries/275a021bbfb6489e54d471899f7db9d1663fc695ec2fe2a2c4538aabf651fd0f" 2016-07-25 5:37:00 PM,335 [lib.cuckoo.core.scheduler] INFO: Task #6: acquired machine fed01 (label=fed01) 2016-07-25 17:37:00,345 [modules.auxiliary.sniffer] INFO: Started sniffer with PID 9360 (interface=virbr0, host=192.168.122.10, pcap=/home/tscherf/cuckoo/cuckoo/storage/analyses/6/dump.pcap) tcpdump: listening on virbr0, link-type EN10MB (Ethernet), capture size 262144 bytes 2016-07-25 17:37:02,801 [lib.cuckoo.core.guest] INFO: Starting analysis on guest (id=fed01, ip=192.168.122.10) 2016-07-25 17:39:14,711 [lib.cuckoo.core.guest] INFO: fed01: analysis completed successfully 31 packets captured 31 packets received by filter 0 packets dropped by kernel 2016-07-25 17:39:16,637 [lib.cuckoo.core.scheduler] INFO: Task #6: reports generation completed (path=/home/tscherf/cuckoo/cuckoo/storage/analyses/6) 2016-07-25 17:39:16,755 [lib.cuckoo.core.scheduler] INFO: Task #6: analysis procedure completed
On the basis of the output, you can easily detect the tool's workflow. After the readme.txt
sample file has been received, a new analysis task can be started and a new virtual machine created from the snapshot created previously. The -machine
option lets you define which virtual machine Cuckoo should use (e.g., if you created several systems previously), because you want to use different operating systems for the analysis.
If the system is running, the file is transferred, and a network sniffer launches to grab the network traffic off the bridge. In this example, the analysis takes about two minutes. Subsequently, Cuckoo publishes the reports in the storage/analyses/<report>/
folder. For this test, I defined in the reporting.conf
file that I wanted to produce an HTML report that could be viewed easily in a web browser (Figure 3).
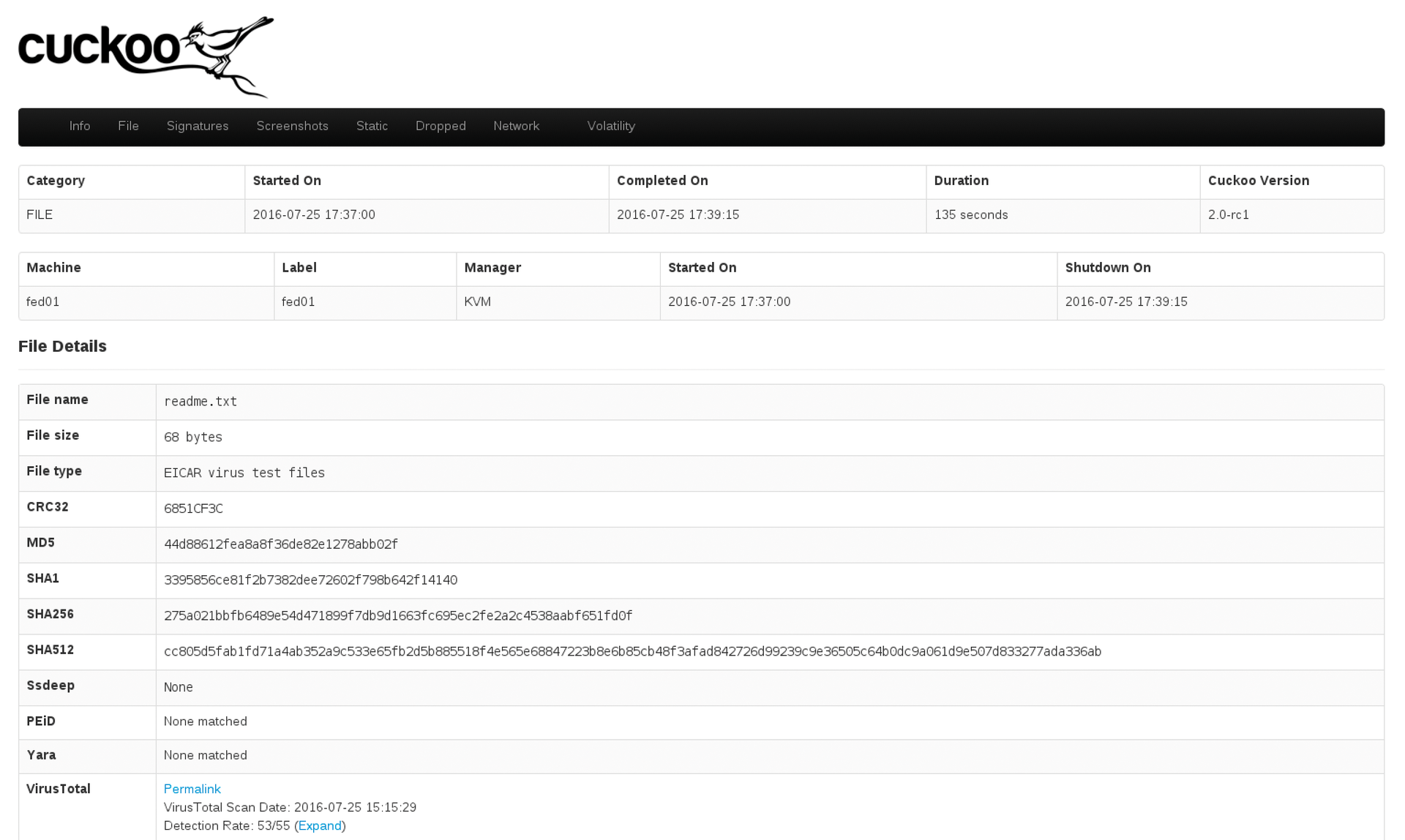
The actual investigation of malware samples is conducted in Cuckoo by the analysis packages residing in the installation directory under analyzer/modules/packages/
. Cuckoo tries to discover which of these packages to use according to the file type. Alternatively, the corresponding analysis package can be stated when uploading the samples. If you are inspecting a PDF file, the call might look like:
$ utils/submit.py --package pdf --machine win01 evil.pdf
Cuckoo can inspect entire websites for defective code. To do so, you need to call the submit.py
tool with the url
option:
$ utils/submit.py --url http://lexu.goggendorf.at/nukgfr2.html
In addition to the tool for uploading malware samples, Cuckoo provides some more interesting utilities. For example, the latest modules for reporting, analyzing, and processing samples can be downloaded with the community.py
tool. The stats.py
tool shows statistics for the completed tasks (Listing 4).
Listing 4: Reporting Modules
$ python utils/community.py --reporting Downloading modules from https://github.com/cuckoosandbox/community/archive/master.tar.gz Installing REPORTING $ python utils/stats.py 4 samples in db 11 tasks in db pending 0 tasks running 0 tasks completed 0 tasks recovered 0 tasks reported 8 tasks failed_analysis 3 tasks failed_processing 0 tasks failed_reporting 0 tasks roughly 0 tasks an hour roughly 1 tasks a day
Professional users of the software will appreciate the Cuckoo API [8], which allows many jobs carried out manually to be automated and integrated with existing tools.
At this point, I should note that Cuckoo can also run in a cluster, which is very useful if you need to run a large number of tasks simultaneously. Because Cuckoo starts a virtual machine for each analysis, running Cuckoo on a single machine does not scale after a certain number of tasks running in parallel; at this point, a cluster setup becomes worthwhile. The software provides a REST API for this purpose that can be used to submit samples. The distributed/app.py
tool then forwards the incoming samples to one of the previously registered Cuckoo nodes. For more information about this topic, check out the quite detailed Cuckoo documentation [8].
Conclusions
Cuckoo is a very powerful tool for analyzing malware. Thanks to the modular implementation, the software can be expanded very easily with your own modules and thus adapts ideally to suit your needs. If you want to take a look at the software before installing, pay a visit to the free malwr malware analysis service [9], which uses Cuckoo as the back end.